Data science in finance: 5 applications transforming the industry
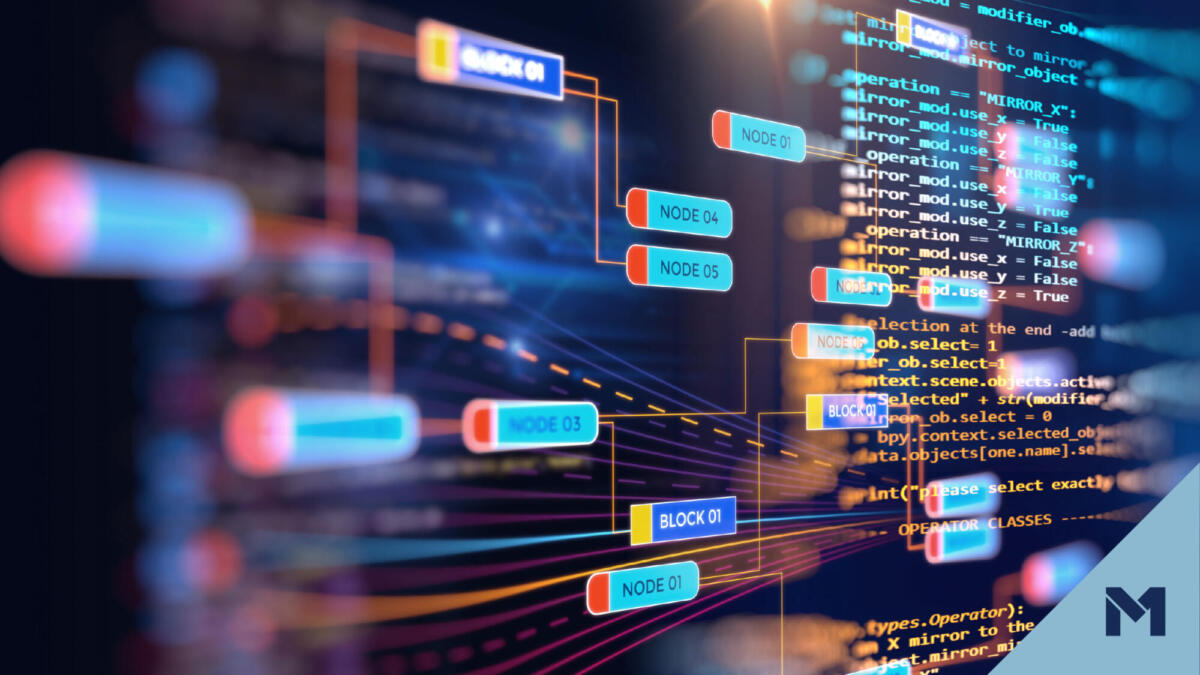
Financial and marketing analysts have been leveraging data to deliver valuable insights and help guide corporate strategies for decades. More recently, finance companies have started to use data and advanced algorithms to uncover trends and predict events that might otherwise remain hidden from analysts.
Data science in the finance industry is transforming core problems such as trading, risk management, and fraud identification. Yet, its impact extends to areas you might not immediately think of, like the ability to acquire and retain high-quality customers. This includes leveraging data science to improve a company’s marketing, products, and services.
5 applications of data science in finance
To better understand the impact of data science in finance, here are five common ways finance companies use it to drive business.
1. Algorithmic trading
The velocity and volume of the financial markets pose significant challenges for people as they attempt to monitor and execute trades. To help resolve this, about 20 years ago, machine learning pioneers began to develop and deploy systems that could quickly process and make sense of the deluge of market data.
Today, these algorithmic trading systems “listen” to broad sets of the market in real-time to find signals that are difficult for analysts to find. These signals predict short-term market movements. Without the need of a human supervisor, the algorithms can automatically execute trades to take advantage of these predicted market movements before they occur.
M1 doesn’t use algorithmic trading, but this practice is common among large institutional investors such as hedge funds. According to Seeking Alpha, algorithmic trading drives a staggering 80% of the market volume in the United States. And a research report by MarketsandMarkets predicts the algorithmic trading market is growing at a rate of 11% each year and will hit $18.8 billion by 2024.
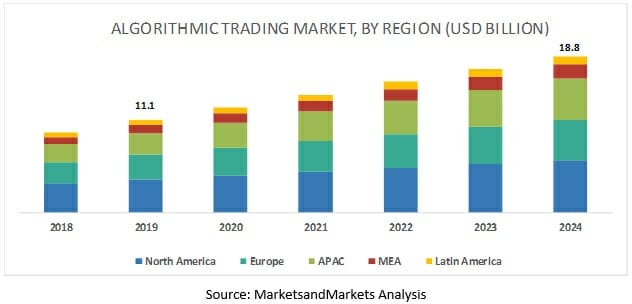
2. Credit assessment
Making credit widely available can be great for individuals and the broader economy because it allows households and businesses to purchase items and services on credit and invest in their futures.
The financial institutions offering this credit earn interest and transaction fees. So it’s a win-win. However, not everyone pays back their debt, which causes financial distress to the debtor, eats into banking profits, and can cascade into broader market consequences (such as the 2007-09 Great Recession).
How does a lending institution know whether to say “yes” or “no” to someone requesting access to financing? Enter the world of credit decisioning, which historically relied on business rules and human decision-makers. However, people might not be able to accurately and fairly assess an applicant’s creditworthiness.
Most lending organizations deploy machine learning (ML) algorithms that sift through past transactions and customer attributes to predict the likelihood a new applicant will repay their debt. Financial companies can also use machine learning to set interest rates for certain products like mortgages or personal loans to reflect default risk. This has numerous benefits because it:
- Cuts the time that an applicant needs to wait to receive a decision
- More efficiently allocates assets that can be lent to those who are more likely to repay
- Decreases human biases that might unfairly discriminate against certain loan applicants
- Frees up credit analysts to conduct other work for the lending organization
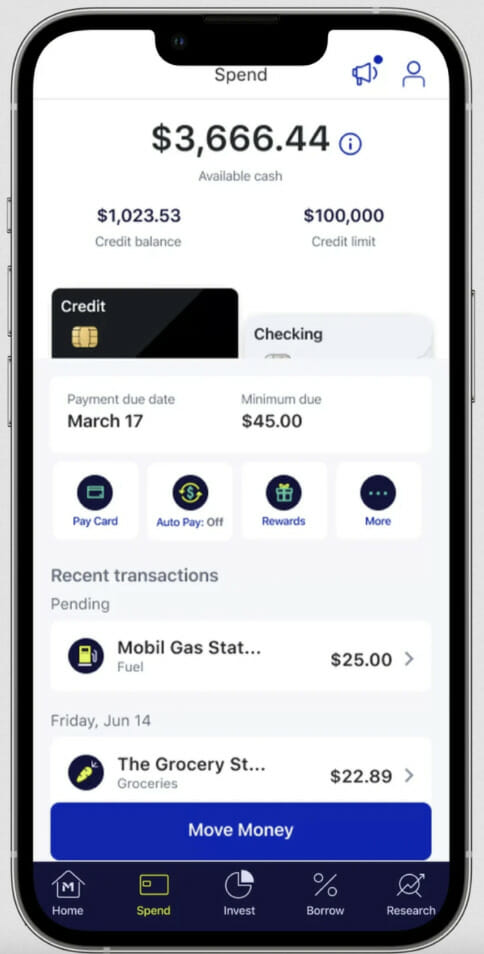
3. Fraud detection
You’ve likely received a call, text, or email from your bank to verify suspicious activity. You might have even had your card blocked or canceled as a result.
Behind the scenes, this is probably the work of a machine learning algorithm. Data scientists can train an ML model on historical user behavior with a fraud target variable. The algorithm identifies key feature values that contribute to fraud detection.
Whenever a transaction occurs on your credit or debit card, the system assesses whether it’s beyond the norm of your activity and whether that behavior tends to match patterns frequently used by fraudsters. If the system sees something fishy, it can send out an automated alert to ask you to verify the transaction or (if deemed of high enough risk) block the transaction before completing the purchase.
Yes, these verifications and holds can be annoying. But they take a bite out of estimated $30+ billion of card fraud losses that occur each year.
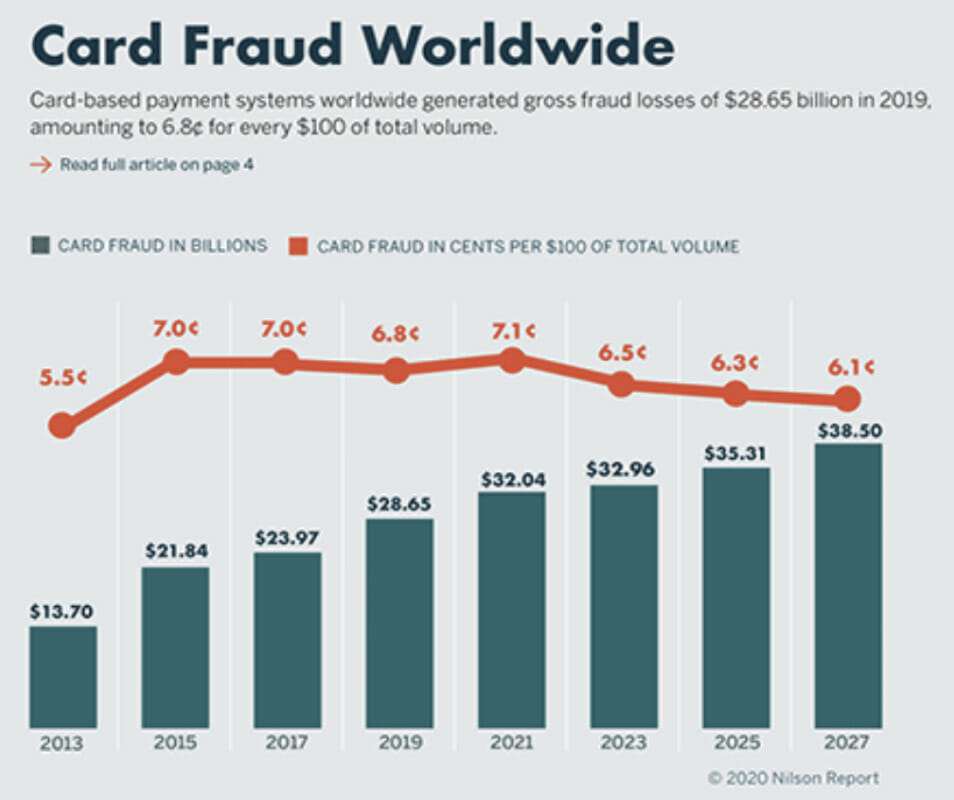
4. Customer lifetime value
You might think, “Wait! Customer lifetime value (LTV) is a marketing use case.”
You’re right. But companies need customers, and many use cases for data science in finance focus on marketing.
How do you know whether a customer is high-quality? Customer lifetime value modeling can help a company predict the value that each customer (or group of customers) provides to the organization. This value is typically a function of the user’s predicted net cashflows—average revenue per user times the profit margin of that revenue—over their tenure as a customer.
simplified LTV = ARPU x profit margin x tenure
In practice, organizations use more complex models to predict each user’s LTV by combining financial assumptions and machine learning propensity models. Finance teams provide assumptions like the discounting factor and profit margin. Meanwhile, data science teams predict customer behavior like churn propensity, the likelihood of a customer engaging in a certain service, or a customer’s expected Assets Under Management.
Collectively, this helps the marketing department answer key questions such as:
- How much should we spend on acquiring a new customer?
- What products and services should we promote?
- Will we hit long-term profitably?
5. Marketing campaign experiments
Marketing teams across finance companies conduct campaigns to increase or decrease certain customer behaviors. This can include:
- Conduct a Google AdWords campaign to get high-quality potential customers to download their app.
- Create an e-mail campaign to encourage existing customers to consider the benefits of retirement accounts.
- Add pop-ups to their website to collect emails of potential customers.
But how do we know whether the campaign led to the target action? A simple way is to observe whether the target action occurred more frequently during or shortly after the campaign than before. This is commonly referred to as pre/post analysis.
However, this approach isn’t ideal because several additional factors impact user behavior. For example, let’s say a finance company like M1 is trying to encourage a user to consider opening a brokerage account. During the observation period, more users funded the account. But a hot new meme stock also took the media by storm. Was it the campaign that led to the increased funding or the meme stock? Or maybe it was some other seasonal or market factors.
To know whether the campaign itself caused users to open accounts, companies need to isolate the intervention and control from other factors. To do this, data science teams can work with marketing to design an experiment.
In the simplest (and often most effective) approach, users are randomly divided into two groups: 1) a treatment group that receives the marketing campaign and 2) a control group that doesn’t receive the communication. After marketing runs the campaign, data scientists and marketing analysts compare the two groups to see whether the treatment group performed statistically better than the control group.
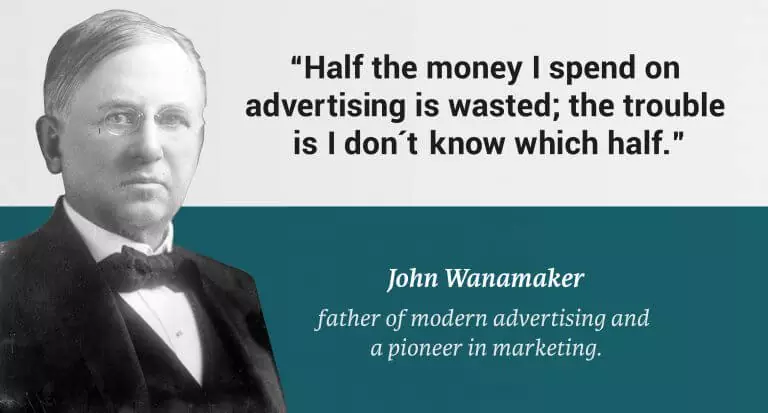
Other use cases for data science in finance
The above applications only scratch the surface of data science in finance. Many sizable companies are using data science in a variety of ways, including:
- Robo-advising – Provides recommendations or automated trades based on efficiently allocating assets to optimize rewards given risk tolerance and investment time horizon.
- Expected loss modelling – Estimates the value of credit products such as loans that are bad.
- Staffing models – Predicts how many people are likely to contact customer service so that the company can staff the department appropriately.
- Chatbots – Automates common responses and mimics human-like interactions via a bot behind a chat messaging system on the finance company’s website or app.
- Text analysis – Uses natural language processing to identify major topical themes in text from online reviews, surveys, and support tickets.
- Uplift modeling – Estimates incremental value that companies get from various activities, such as calling specific customers.
- Discrimination reduction – Detects whether unfair decisions are being made against specific protected classes of people.
- Price sensitivity analyses – Predicts the change in the number of customers who subscribe to services with increases or decreases in the service price.
The future of data science in finance
Data science adoption in finance is accelerating, and several factors are driving this growth.
- The quality and quantity of data being collected and available for purchase is increasing.
- The pool of data professionals is growing as professionals are attracted to data roles due to strong job satisfaction, job growth, and high wages.
- The tight labor market and wage inflation is driving companies to look for ways to automate routine tasks.
- Data science tools—both open source and proprietary—are maturing and becoming more widely available to non-technical users.
- Finance leaders are increasingly encouraging their teams to leverage data-driven decisions.
- Fintech companies like M1 are challenging conventional decision-making approaches. This increased competition is forcing other fintechs and traditional finance companies to innovate.
The data science field is growing quickly across business verticals, and the financial industry is already experiencing its impact. As technology continues to improve, data availability increases, and senior leads realize data’s value, data science will further drive finance companies to offer better, more secure, and fairer products and services to end users.
Learn how M1 uses data and automation to help you invest >>
About the author: Nicholas Hotz is a Data Science & Analytics Product Manager at M1.
- Categories
- Plan