Building a centralized analytics organization through a decentralized divisional model
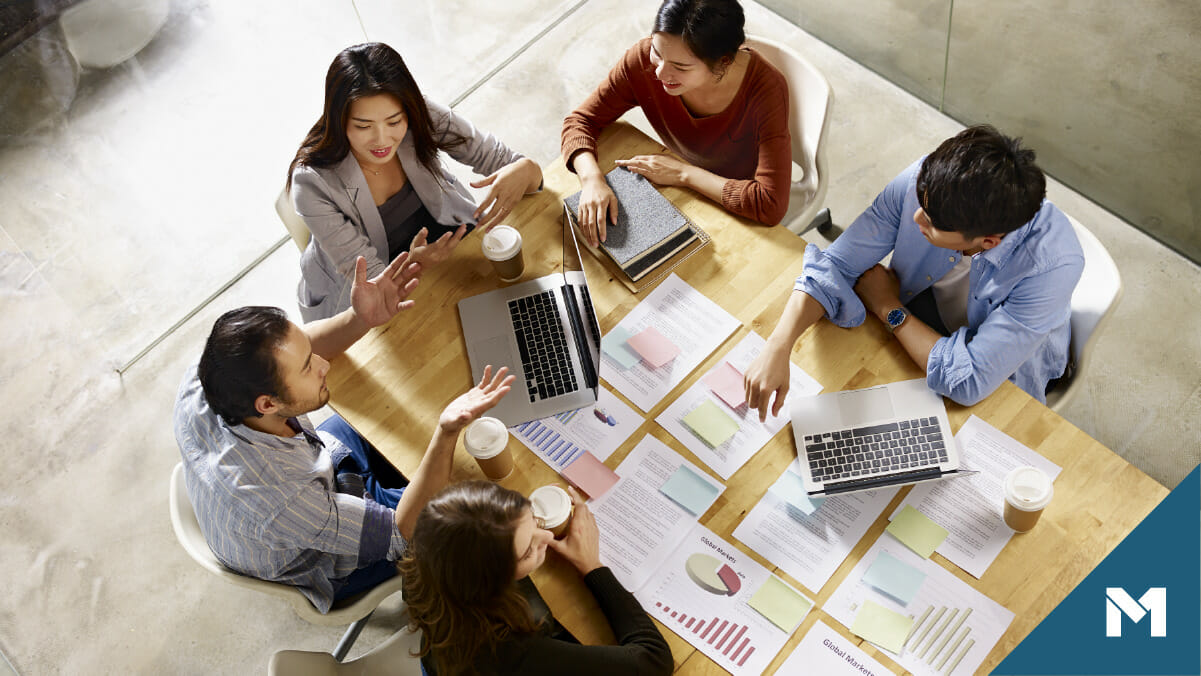
In early 2021, M1 had roughly 100 team members—two of which made up the analytics team. At that size, managing a central backlog of requests for analysis was “relatively” easy. The marketing team was the primary stakeholder, and everyone aligned on priorities in a single meeting.
At the time, our analytics work intake pattern was like many service organizations.
Stakeholders requested a dashboard, report, or analysis either through Slack, in a meeting, or via a form. We added the request to the backlog. Every two weeks, we met with stakeholders to discuss which requests to prioritize. Once an analyst finished their existing work, they would get new work assigned.
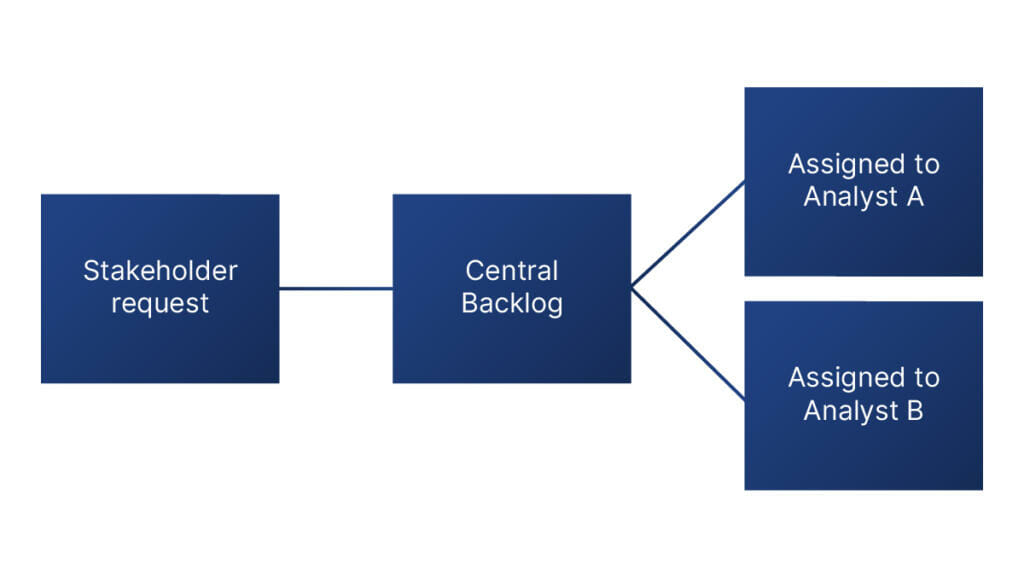
This model works relatively well when a team is small and stakeholders’ objectives are closely aligned. So, we kept this approach as M1 grew from 100 to 200 employees. Soon, the complexity of the stakeholders ranged from marketing and business strategy to accounting and finance.
We positioned our product to meet clients’ specific needs, but it complicated our prioritization problem in analytics.
Best-in-class financial products, not just in one place, but made better because of the place, so that the whole is greater than the sum of its parts.
How do you choose between which product to support when the goal is to make them all best-in-class? One solution to this is to simply separate each product division and let them build out their own analytics function. This happens a lot at larger corporations where each division acts as its own business.
The downside of this approach is the loss of symbiosis across the analytics teams. This often manifests in an odd tech stack, where the organization may have three different BI tools because each division’s analytics team purchases their own tools. Similarly, it creates silos that wall off analysts from other divisions. The approach may enable best in class siloed products, but it misses the synergic components where the “whole is greater than the sum of its parts”.
We chose a different path.
At M1, we deploy a division and specialization model.
What are divisions?
Divisions are a separation of teams that align to a specific product. The division determines its data needs and is ultimately responsible for prioritization. For example, the “M1 Invest” division focuses on improving our M1 Invest product and requires dedicated analytics team members.
What are specializations?
Specializations are the functional specialties that determine direct-reporting relationships for analysts. For example, multiple marketing analysts who support different divisions report into a single marketing analytics manager.
Although the divisions operate and prioritize analyses autonomously, this structure helps our analysts maintain consistent standards within a given specialization (e.g., marketing analytic) across divisions.
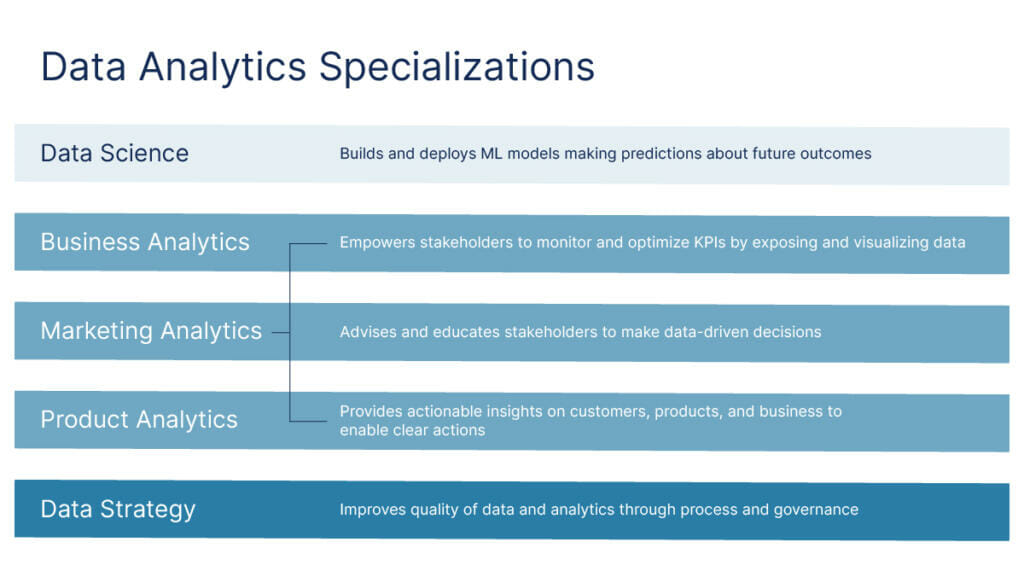
Specializations allow analysts to tailor the hiring and team development processes to domain-specific expertise, like product clickstream analytics versus marketing campaign analysis.
At scale the model looks like this:
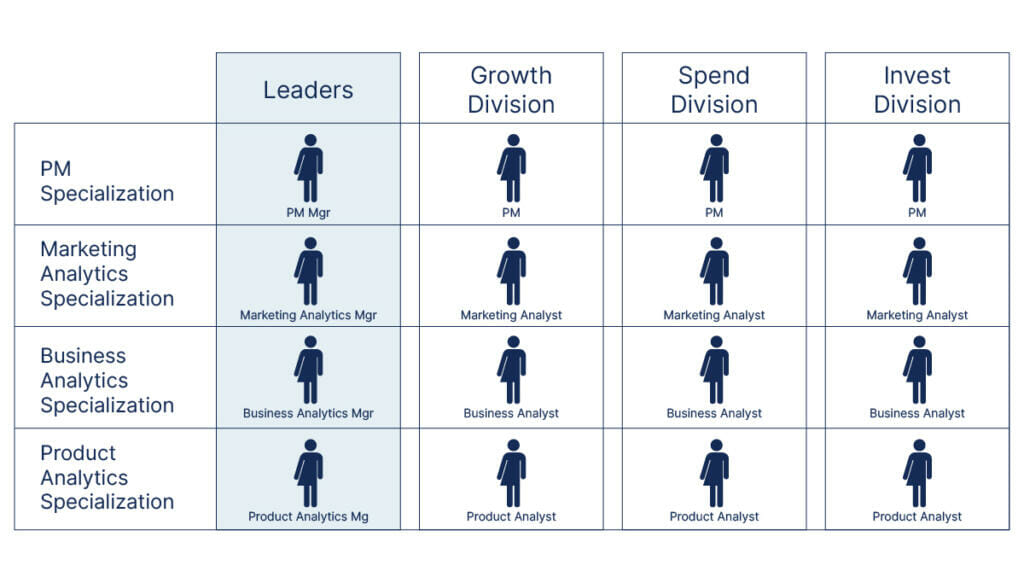
Each analyst reports to a leader and is part of a team supporting a specific division. The model is flexible in that one analyst could support multiple divisions, switch divisions, or gain exposure to multiple specializations. This is useful as companies add new divisions over time.
At M1, an analyst may start in business analytics supporting our M1 Spend product but then move to support the Growth division as a marketing analyst.
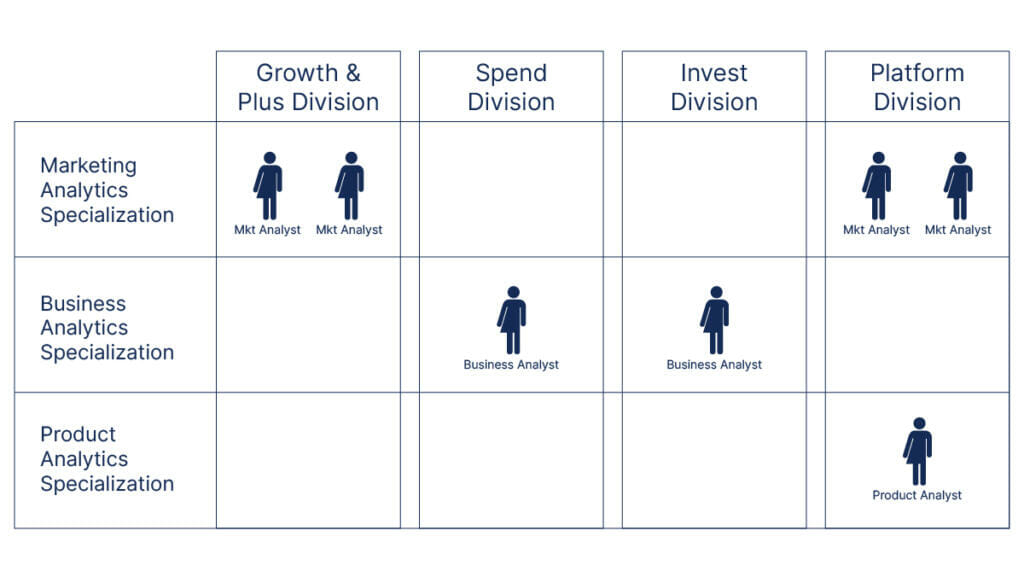
Working on a breadth of products gives analysts the opportunity to deepen their domain expertise as a whole or within a specific domain.
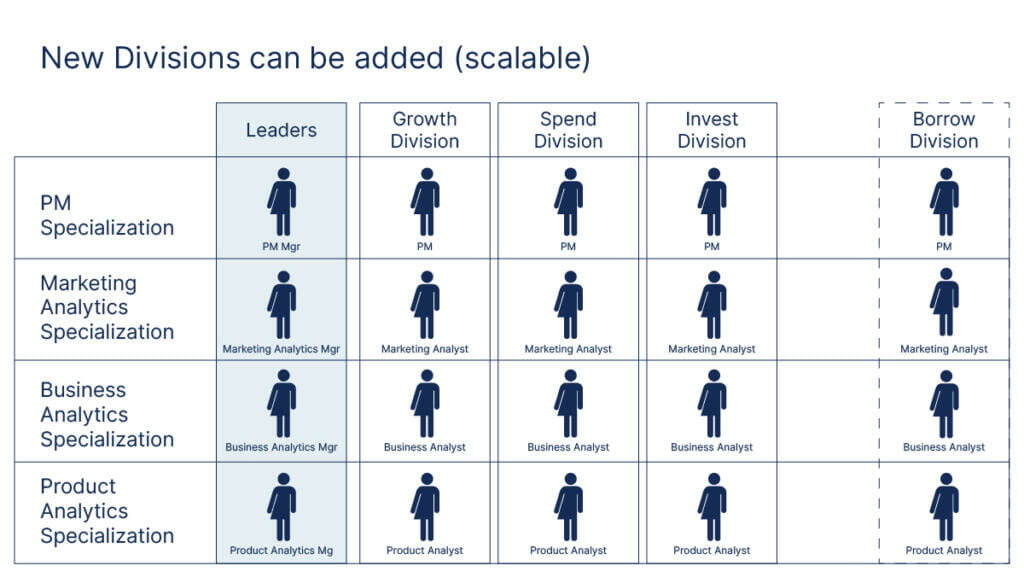
As business needs evolve to require new expertise, analysts can also add new specialization and deepen exposure to analytics concepts.
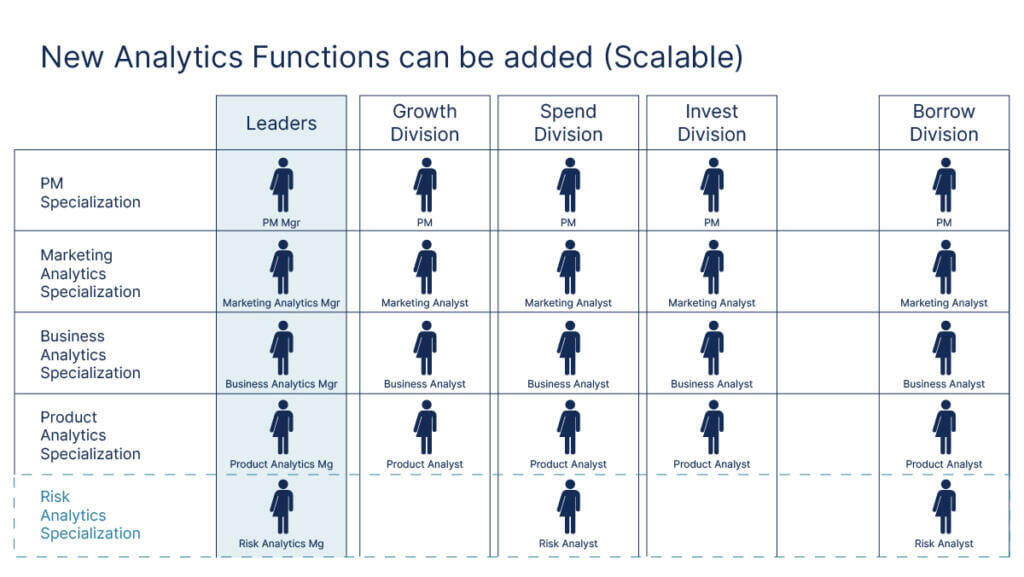
While a division’s team size shouldn’t grow too large, the model doesn’t require a specific number of analysts per specialization. Some areas may require more marketing analysts, where others may need more product analytics expertise.
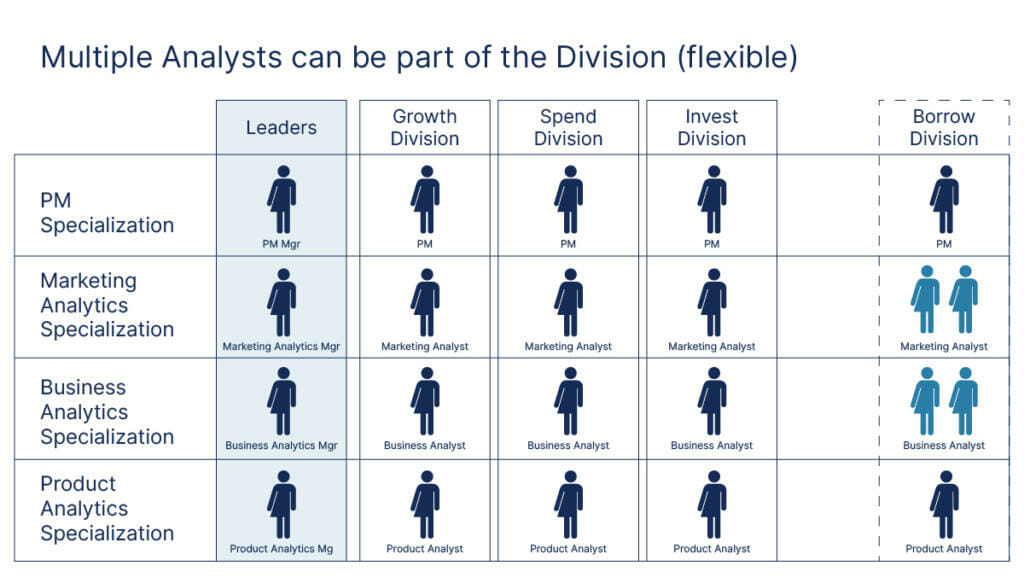
The divisional model is much less formal than a large, centralized backlog.
Each division team follows their own process in terms of meeting cadence and prioritization framework. This gives greater flexibility when it comes to how divisions work and make decisions. Instead of requiring divisions to change how they work, it aligns people with each other and drives towards individual divisional outcomes—and it adheres to analytics standards.
The divisional model encourages autonomy and creativity by trusting people to complete their work in the way they see fit.
Do we need to ship a dashboard? That’s up to the division. Do we need to change direction? That’s also up to the division. The model focuses on decentralizing decision making and transferring that responsibility to divisions.
By implementing this model, our analysts are more aligned to the business and reap the benefits of being part of an analytics organization that using data science to improve finance. This can lead to better data products, happier stakeholders, and more insightful analysis.
Learn more about the product that inspired this divisional model, M1 Invest.
About the author: Nicklas Ankarstad is the Senior Director of Data Science and Analytics at M1.
- Categories
- M1